- Sijie Song
ssj940920@pku.edu.cn
- Cuiling Lan  
culan@microsoft.com
- Junliang Xing
jlxing@nlpr.ia.ac.cn
- Wenjun Zeng
wezeng@microsoft.com
- Jiaying Liu
liujiaying@pku.edu.cn
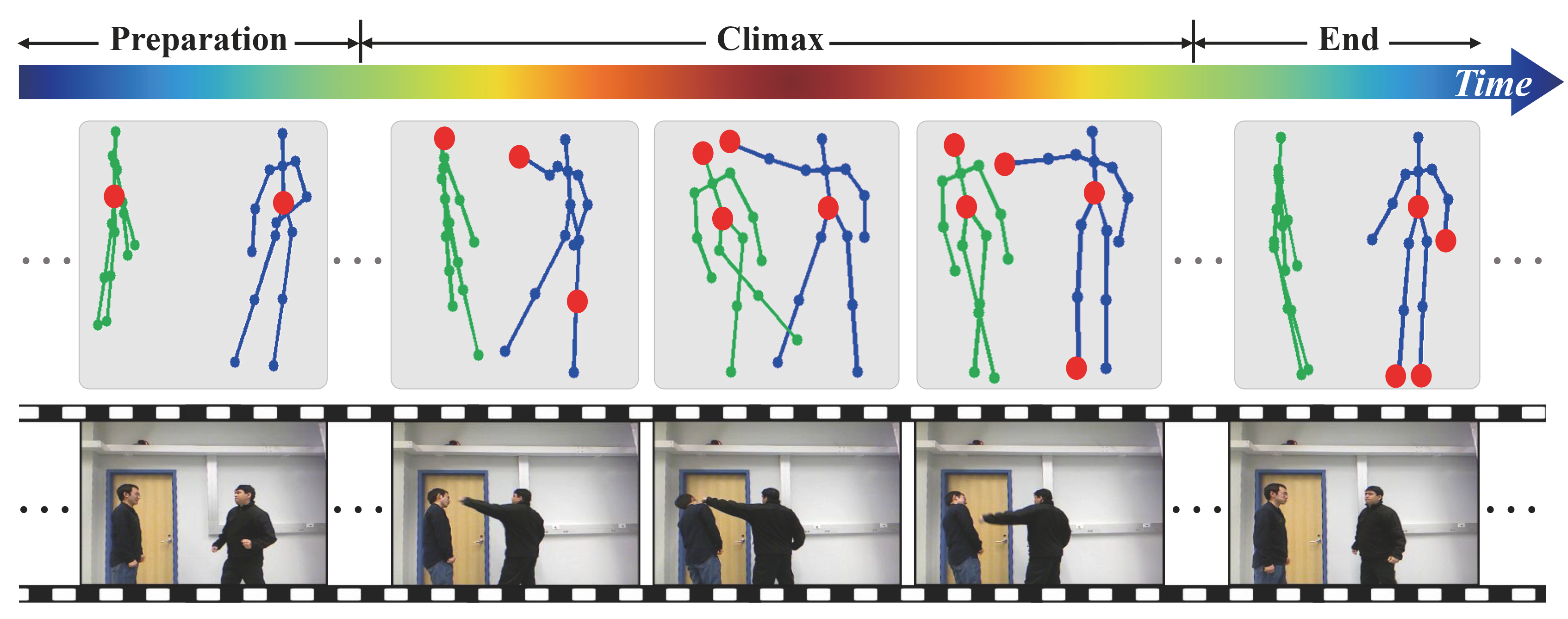
Fig.1. Illustration of the procedure for an action "punching". An action may experience different stages, and involve different discriminative subsets of joints (as the red circles).
Abstract
Human action recognition is an important task in computer vision. Extracting discriminative spatial and temporal features to model the spatial and temporal evolutions of different actions plays a key role in accomplishing this task. In this work, we propose an end-to-end spatial and temporal attention model for human action recognition from skeleton data. We build our model on top of the Recurrent Neural Networks (RNNs) with Long Short-Term Memory (LSTM), which learns to selectively focus on discriminative joints of skeleton within each frame of the inputs and pays different levels of attention to the outputs of different frames. Furthermore, to ensure effective training of the network, we propose a regularized cross-entropy loss to drive the model learning process and develop a joint training strategy accordingly. Experimental results demonstrate the effectiveness of the proposed model, both on the small human action recognition dataset of SBU and the currently largest NTU dataset.
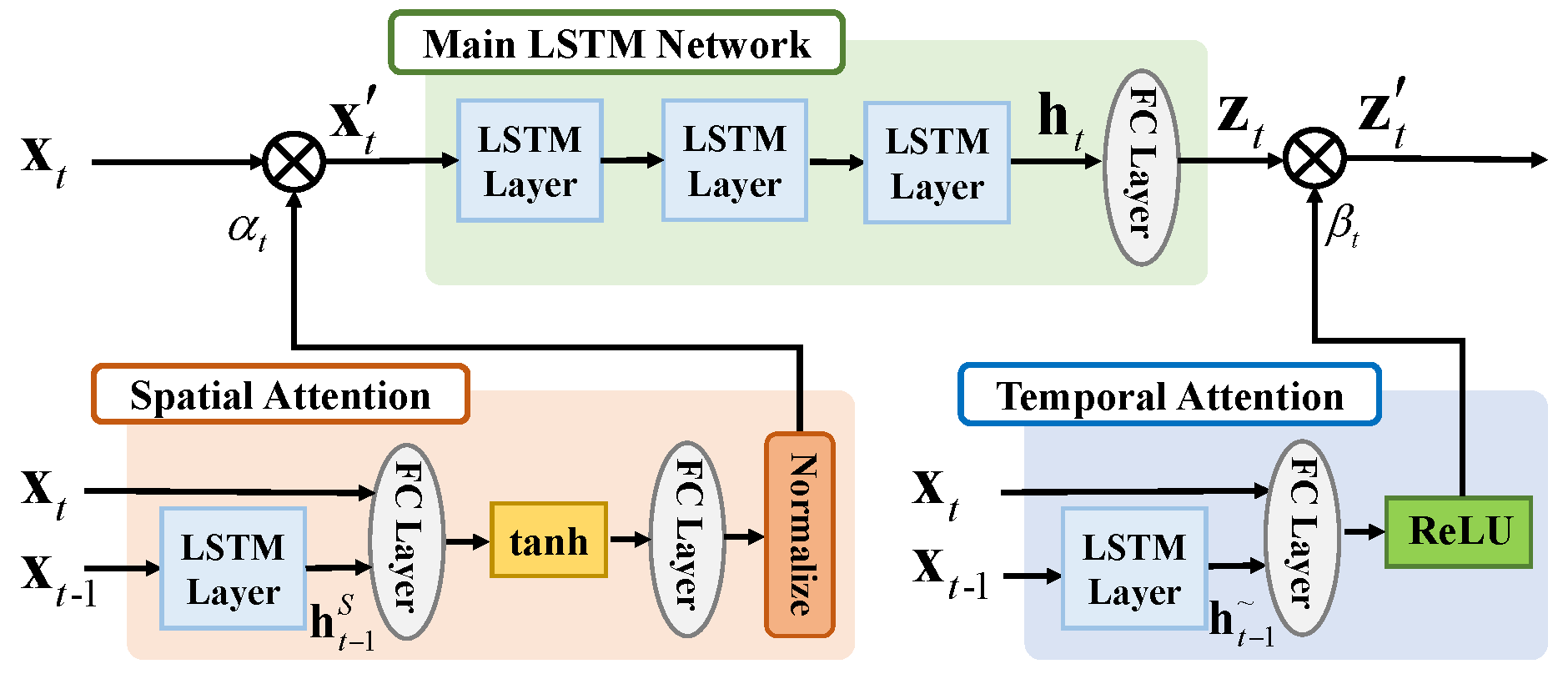
Fig.2. The overall architecture of our method.
Visualization of temporal and spatial attention
Here we show samples to visualize the temporal and spatial attention. We mark the 8 joints with the largest attention weights using red circles in skeleton. And the upper in the right bottom figure shows the attention weights of the left actor, while the bottom shows those of the right actor.
Video 1. Kicking
Video 2. Pushing
Download
Citation
@InProceedings{song2016end,
title={An End-to-End Spatio-Temporal Attention Model for Human Action Recognition from Skeleton Data},
author={Song, Sijie and Lan, Cuiling and Xing, Junliang and Zeng, Wenjun and Liu, Jiaying},
booktitle ={AAAI Conference on Artificial Intelligence},
year={2017},
pages={4263--4270}
}