- Sijie Song
ssj940920@pku.edu.cn
- Yanghao Li  
lyttonhao@gmail.com
- Zhihan Gao
gaozhihan@pku.edu.cn
- Jiaying Liu
liujiaying@pku.edu.cn
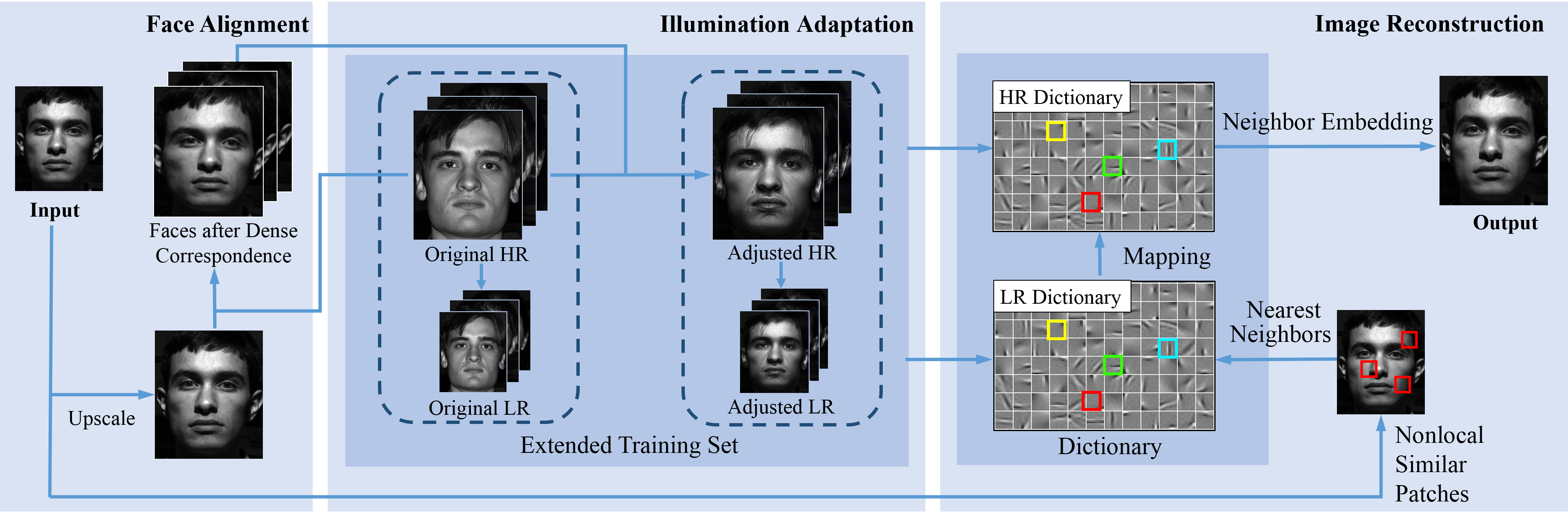
Fig.1. The Framework of the Face Hallucination Based on Neighbor Embedding via Illumination Adaptation.
Abstract
In this paper, we present a novel face hallucination method by neighbor embedding considering illumination adaptation (NEIA) to super-resolve faces when the lighting conditions of the training faces mismatch those of the testing face. For illumination adjustment, face alignment is employed through dense correspondence. Next, every training face is composed into two layers to extract both details and highlight components. By operating the two layers of each face respectively, an extended training set is acquired by combining the original and adapted faces compensated in illumination. Finally, we reconstruct the input faces through neighbor embedding. To improve the estimation of neighbor embedding coefficients, nonlocal similarity is taken into consideration. Experimental results show that the proposed method outperforms other state-of-the-art methods both in subjective and objective qualities.
Experimental Results
We use the Extend Yale Face Database B [1] to evaluate the proposed method. One set under the same lighting condition 000E+00 is utilized as the training set. And we use another four testing sets under different illumination conditions (+000E+45, +005E+10, +015E+20, +035E+15) as the testing face. The difference between the five illumination conditions can be viewed in Fig.2.
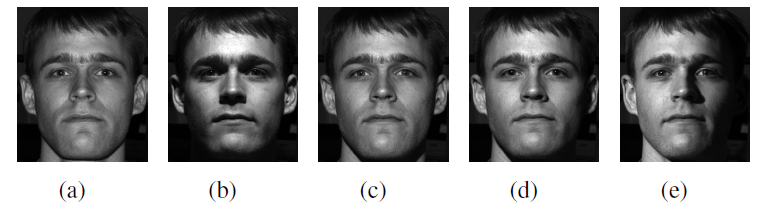
Fig.2. Comparison of different illumination conditions. (a) +000E+00, (b) +000E+45, (c) +005E+10, (d) +015E+20, (e)+035E+15.
Effectiveness of Illumination Adaptation
To confirm the effectiveness of illumination adaptation (IA), we impletement the same neighbor embedding algorithm but with different training sets. The only difference of the training sets is whether it is processed by IA or not. The effectiveness of IA can be proved from the zoomed comparison of the highlighted part as shown in Fig.3 below.It is obvious that the egde of the ear is clearer and the highlight in the eyeball is better recovered.
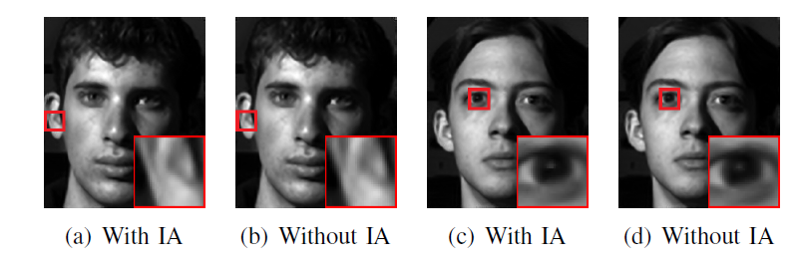
Fig.3. Effecitveness of the illumination adaptation (IA). (a)(c) Results of our method with IA. (b)(d) Results of our method without IA.
Objective Results
In the experiments, we compare the proposed algorithm with the baseline Bicubic method, the position method (PFH) [2], the structured face hallucination method (SFH) [3], the face hallucination method for facial components (NEFC) [4]. Besides, to prove the influence of illumination adjustment, NEIA is also compared with our neighbor embedding method but no illumination adaptation (NENIA). The objective results of four testing sets under different lighting conditions are measured by Peak Signal to Noise Ratio (PSNR) in Table.1. Our proposed method outperforms other methods and achieves the highest PSNR results.
Table 1. Averaged PSNR (dB) results on 4 testing sets (scaling factor = 4)
Testing Set | Bicubic | PFH[2] | SFH[3] | NEFC[4] | NENIA | NEIA | Gain vs. NEFC | Gain vs. NENIA |
---|---|---|---|---|---|---|---|---|
000E+45 | 32.74 | 30.31 | 26.92 | 33.71 | 33.92 | 34.01 | 0.30 | 0.09 |
005E+10 | 32.95 | 31.05 | 27.80 | 34.40 | 34.35 | 34.45 | 0.05 | 0.10 |
015E+20 | 32.53 | 30.35 | 27.07 | 33.88 | 33.87 | 33.98 | 0.10 | 0.11 |
035E+15 | 32.23 | 30.06 | 27.08 | 33.56 | 33.55 | 33.69 | 0.13 | 0.14 |
Average | 32.62 | 30.44 | 27.22 | 33.89 | 33.92 | 34.03 | 0.14 | 0.11 |
Subjective Comparison of Different Methods
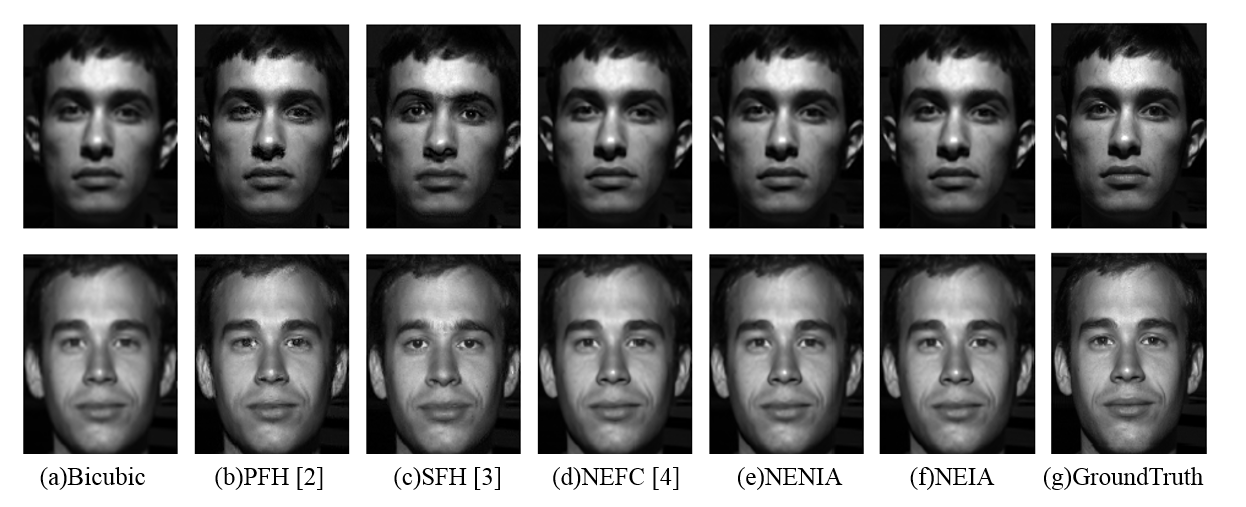
Fig.4. The visual results on two face images under the illumination conditions +000E+45, +005E+10, respectively. (scaling factor = 4)
References
[1] A. S. Georghiades, P. N. Belhumeur, and D. J. Kriegman. From few to many: illumination cone models for face recognition under variable lighting and pose. IEEE Transactions on Pattern Analysis and Machine Intelligence (PAMI), vol. 23, no. 6, pp. 643–660, June 2001.
[2] X. Ma, J. Zhang, and C. Qi. Hallucinating face by position-patch. Pattern Recognition (PR), vol. 43, no. 6, pp. 2224–2236, June 2010.
[3] C.-Y. Yang, S. Liu, and M.-H. Yang. Structured face hallucination. In Proc. IEEE Int’l Conf. Computer Vision and Pattern Recognition (CVPR), pp. 1099–1106, June 2013.
[4] Y. Li, J. Liu, W. Yang, and Z. Guo. Multi-pose face hallucination via neighbor embedding for facial components. In Proc. IEEE Int’l Conf. Image Processing (ICIP), 2015.