Reference Guided Deep Super-Resolution via Manifold Localized External Compensation
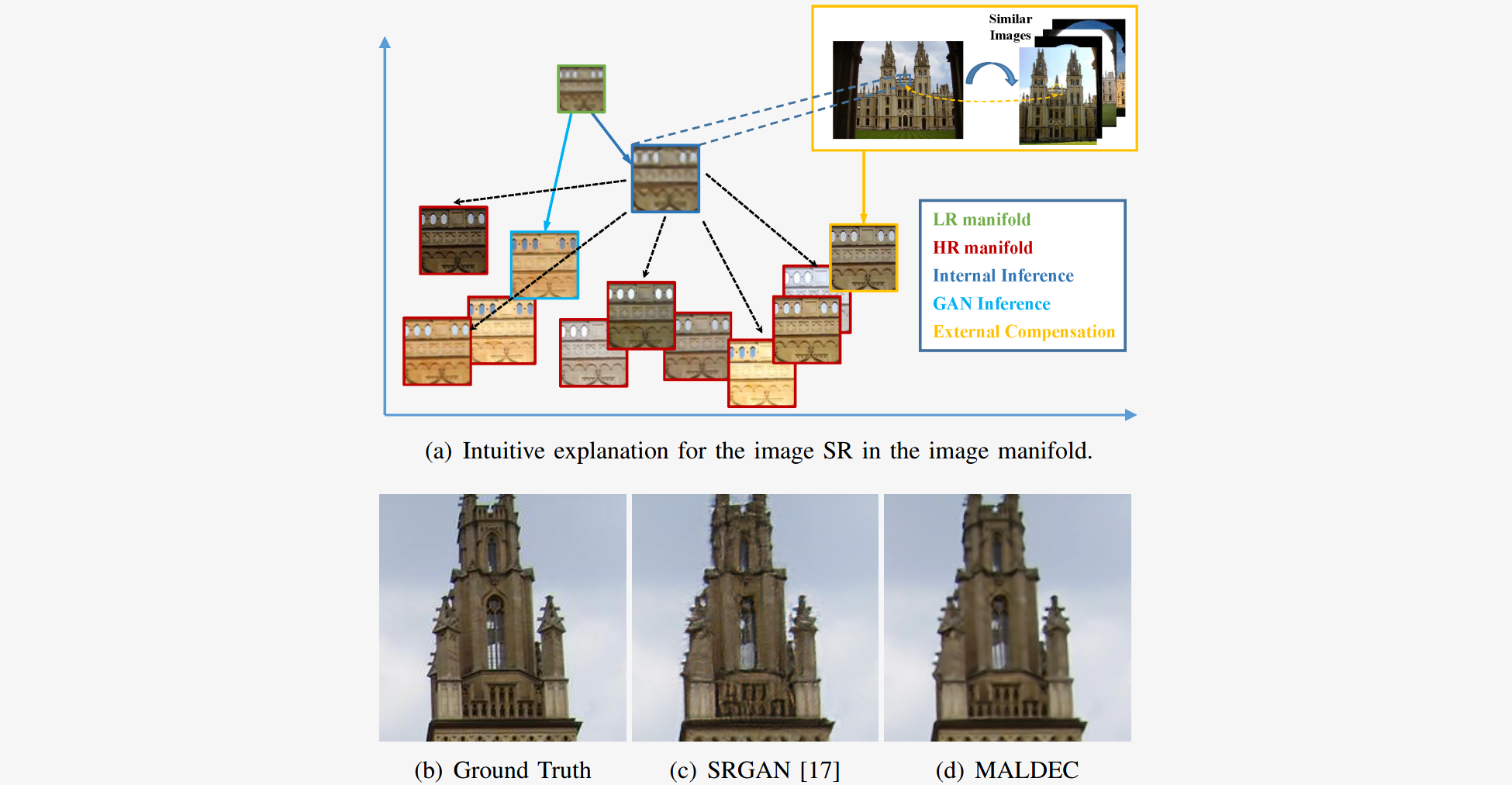
Figure. 1. Internal methods "regress to mean" [Semantic] and generategenerally smooth estimation. GAN drives the reconstructiontowards natural image manifold but presents pixel-level detailartifacts. MALDEC generates both perceptually convincingand structurally promising results.
Abstract
The rapid development of social network and online multimedia technology makes it possible to address traditional image and video enhancement problems, with the aid of online similar reference data. In this paper, we tackle the problem of super-resolution (SR) in this way, specifically aiming to handle the "one-to-many" problem between the image patches of low resolution (LR) and high resolution (HR). We propose a MAnifold Localized Deep External Compensation (MALDEC) network to additionally utilize reference images, i. e. retrieved similar images in cloud database and reference HR frame in a video, to provide an accurate localization and mapping to the HR manifold,and compensate the lost high-frequency details. The proposed network employs a three-step recovery: 1) internal structure inference, which uses the LR image itself and the internally inferred high frequency information to preserve main structureof the HR image; 2) manifold localization, which localizes the HR manifold and constructs the correspondence between the internal inferred image and the external images; 3) external compensation, which introduces the external references of retrieved similar patches based on manifold localization information to reconstruct the high frequency details. The learnable components of MALDEC, internal structure inference and external compensation, are trained jointly to make a good trade-off between these two terms for an optimal SR result. Finally, the proposed method is examined under three tasks: cloud-based image SR,multi-pose face reconstruction and reference frame-guided videoSR. Extensive experiments demonstrate the superiority of ourmethod than state-of-the-art SR methods in both objective and subjective evaluations, and our method offers new state-of-the-art performance.
Framework
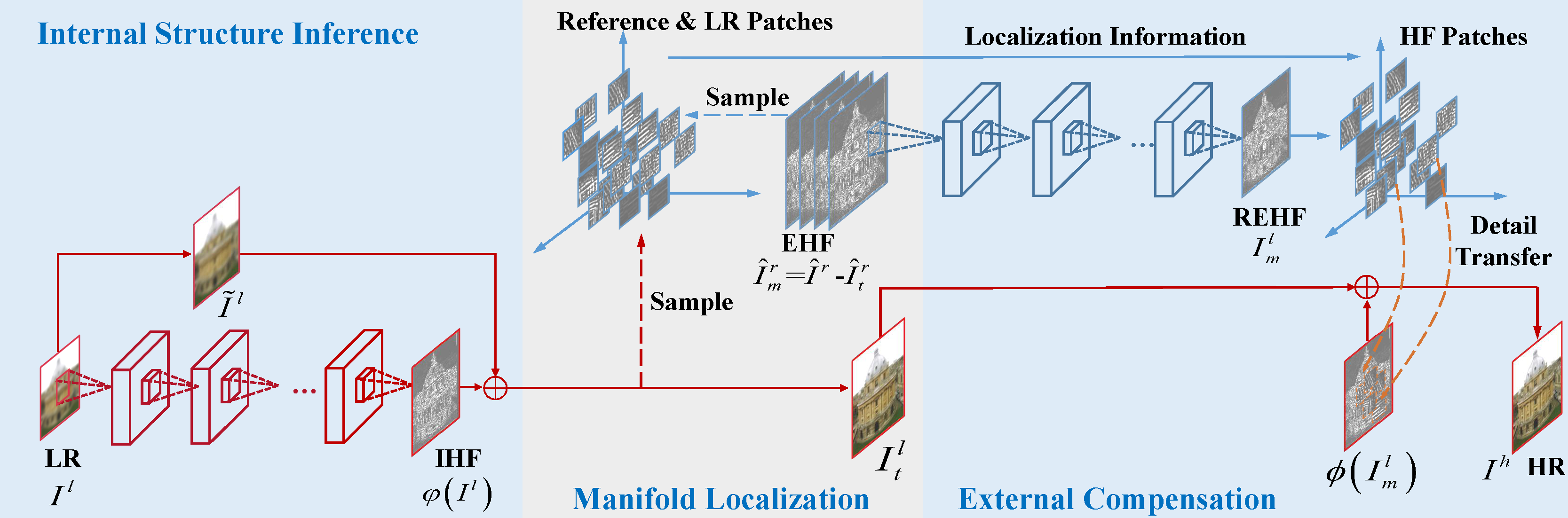
Figure. 2. Framework of the proposed three-step MALDEC to perform joint internal structure inference and external compensation guided by manifold localization. The internal structure inference network (ISIN) learns to predict the HR image as accuratelyas possible solely based on an LR image. In manifold localization, we measure the similarity between the reference patchesand internally inferred patches, and construct the correspondences between them. The external compensation network (ECN)makes up residual lost high-frequency details with the retrieved HR references and manifold localization guidance. IHF, EHF,and REHF signify internal, external, and refined external maps, respectively.
Results
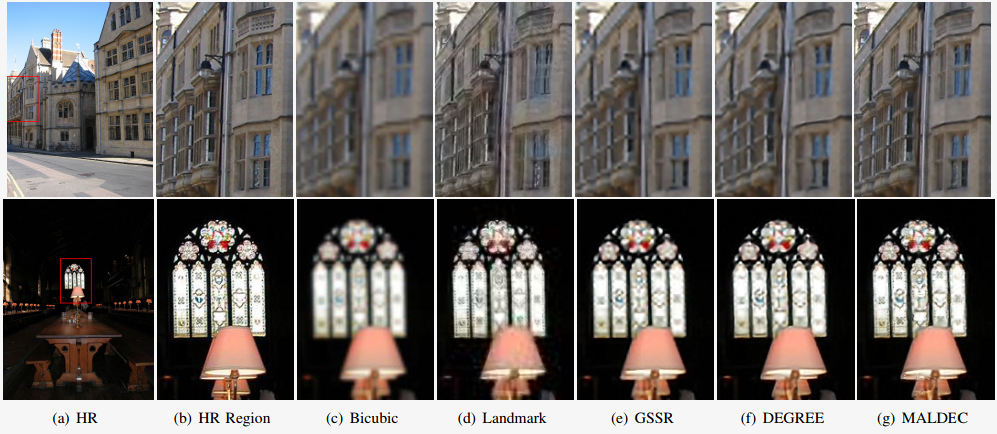
Figure. 3. Visual comparisons of different methods on cloud-based image SR (Configuration I). Top panel: in 4\(\times\) enlargement, MALDEC successfully reconstructs textures of the windows with fewest artifacts. Bottom panel: in 3\(\times\) enlargement, MALDEC generates the most clear and visually pleasing decorations on the window.
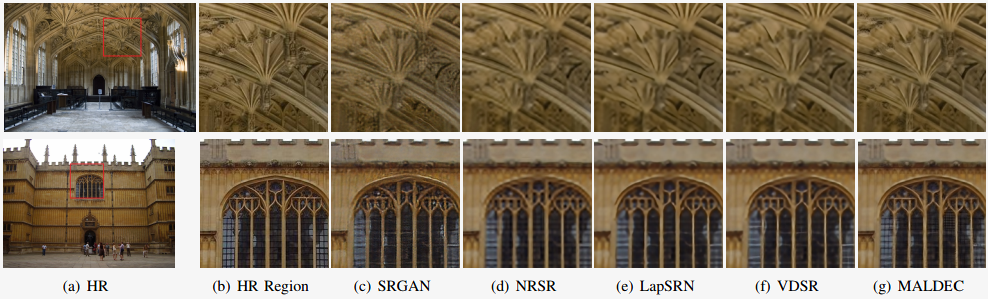
Figure. 4. Visual comparisons of different methods on cloud-based image SR (Configuration II). Top panel: in 2\(\times\) enlargement, MALDEC reconstructs the clearest decorations of the ceiling. Bottom panel: in 4\(\times\) enlargement, MALDEC generates the most clear and visually pleasing details of the window.
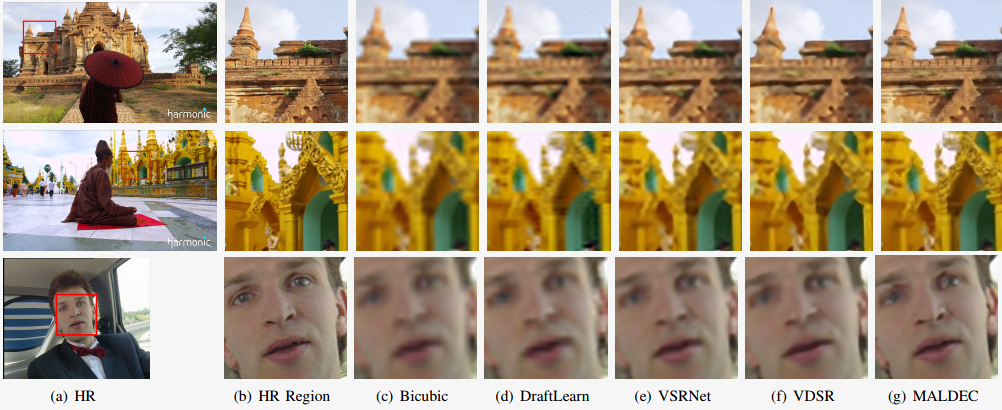
Figure. 5. Visual comparisons of different methods on reference frame-guided video SR (Configuration II). Top two panels: in 4\(\times\) enlargement, MALDEC reconstructs the most detailed decorations of the roofs and walls. Bottom panel: in 4\(\times\) enlargement, MALDEC generates the clearest face details.
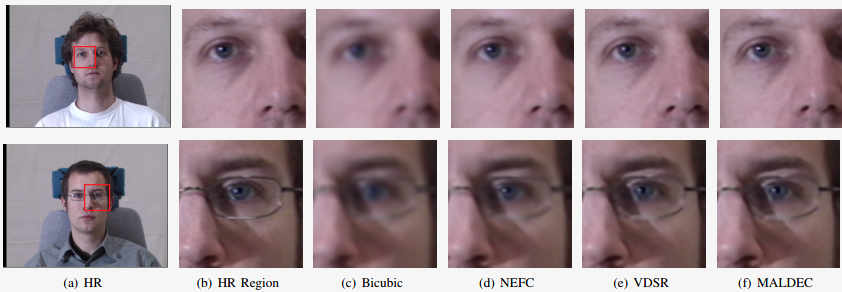
Figure. 6. Visual comparisons of different methods on multi-pose face hallucination (Configuration II). Top panel: 2\(\times\) enlargement. Bottom panel: 3\(\times\) enlargement. MALDEC reconstructs the clearest facial details.
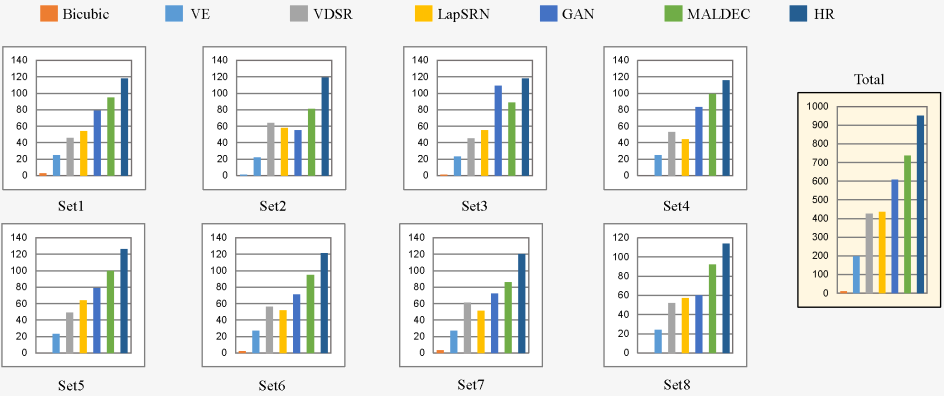
Figure. 7. The number of votes per testing image and the total ranking of seven methods.
Citation
@ARTICLE{8361037, author={W. Yang and S. Xia and J. Liu and Z. Guo}, journal={IEEE Transactions on Circuits and Systems for Video Technology}, title={Reference Guided Deep Super-Resolution via Manifold Localized External Compensation}, year={2018}, volume={}, number={}, pages={1-1}, doi={10.1109/TCSVT.2018.2838453}, ISSN={1051-8215}, month={}, }
Reference
[Semantic] R. Timofte, VD. Smet, and LV. Gool, "Semantic super-resolution: When and where is it useful?," Computer Vision and Image Understanding, vol. 142, pp. 1–12, 2016.
[Landmark] H. Yue, X. Sun, J. Yang, and F. Wu, "Landmark image super-resolution by retrieving web images," IEEE Transactions on Image Processing, vol. 22, no. 12, pp. 4865–4875, December 2013.
[NRSR] Yanghao Li, Jiaying Liu, Wenhan Yang and Zongming Guo. "Neighborhood Regression for Edge-Preserving Image Super- Resolution", Proc. of IEEE International Conference on Acoustics, Speech, and Signal Processing (ICASSP), Brisbane, Australia, Apr. 2015.
[GSSR] J. Liu, W. Yang, X. Zhang, and Z. Guo, "Retrieval compensated group structured sparsity for image super-resolution," IEEE Transactions on Multimedia, vol. 19, no. 2, pp. 302–316, 2017
[DEGREE] W. Yang, J. Feng, J. Yang, F. Zhao, J. Liu, Z. Guo, and S. Yan, "Deep edge guided recurrent residual learning for image super-resolution," IEEE Transactions on Image Processing, vol. 26, no. 12, pp. 5895–5907, Dec 2017.
[SRGAN] C. Ledig et al., "Photo-Realistic Single Image Super-Resolution Using a Generative Adversarial Network," IEEE Conference on Computer Vision and Pattern Recognition (CVPR), Honolulu, HI, 2017, pp. 105-114.
[LapSRN] Wei-Sheng Lai, Jia-Bin Huang, Narendra Ahuja, Ming-Hsuan Yang, "Deep Laplacian Pyramid Networks for Fast and Accurate Super-Resolution," IEEE Conference on Computer Vision and Pattern Recognition (CVPR), Honolulu, HI, 2017, pp. 5835-5843.
[VDSR] J. Kim, J. K. Lee and K. M. Lee, "Accurate Image Super-Resolution Using Very Deep Convolutional Networks," IEEE Conference on Computer Vision and Pattern Recognition (CVPR), June 2016, pp. 1646-1654.
[DraftLearn] R. Liao, X. Tao, R. Li, Z. Ma, and J. Jia, “Video super-resolution via deep draft-ensemble learning,” in Proc. IEEE Int’l Conf. Computer Vision, Dec 2015, pp. 531–539.
[VSRNet] A. Kappeler, S. Yoo, Q. Dai, and A. K. Katsaggelos, “Video superresolution with convolutional neural networks,” IEEE Transactions on Computational Imaging, vol. 2, no. 2, pp. 109–122, June 2016.
[NEFC] Yanghao Li, Jiaying Liu, Wenhan Yang and Zongming Guo. "Multi-Pose Face Hallucination via Neighbor Embedding for Facial Components", Proc. of IEEE International Conference on Image Processing (ICIP), Quebec City, Canada, Sep. 2015.