Demystifying Neural Style Transfer
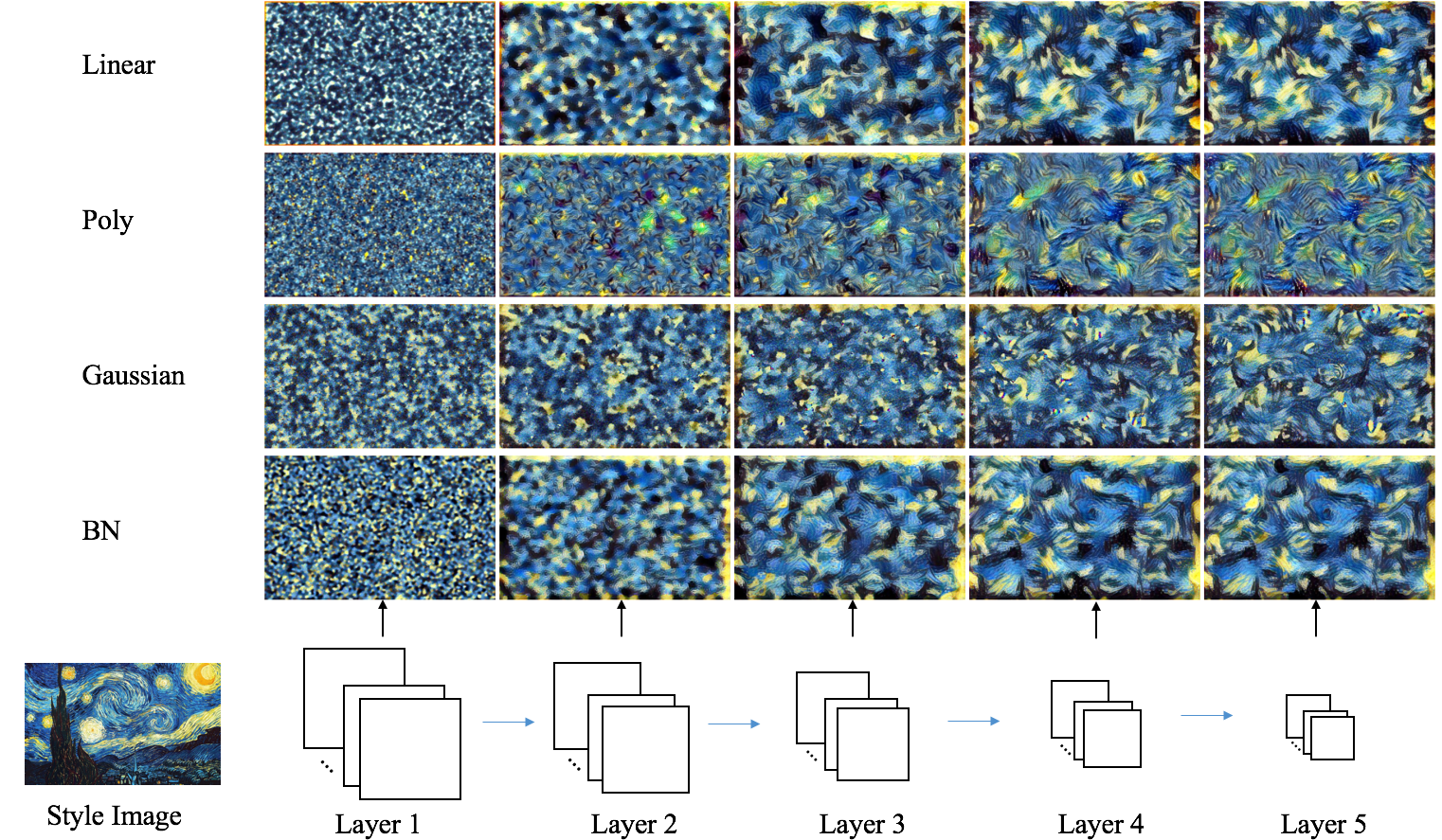
Fig.1 Style reconstructions of different methods in five layers, respectively.
Abstract
Neural Style Transfer has recently demonstrated very exciting results which catches eyes in both academia and industry. Despite the amazing results, the principle of neural style transfer, especially why the Gram matrices could represent style remains unclear. In this paper, we propose a novel interpretation of neural style transfer by treating it as a domain adaptation problem. Specifically, we theoretically show that matching the Gram matrices of feature maps is equivalent to minimize the Maximum Mean Discrepancy (MMD) with the second order polynomial kernel. Thus, we argue that the essence of neural style transfer is to match the feature distributions between the style images and the generated images. To further support our standpoint, we experiment with several other distribution alignment methods, and achieve appealing results. We believe this novel interpretation connects these two important research fields, and could enlighten future researches.
Recourses
Citation
@article{li2017demystifying, title={Demystifying Neural Style Transfer}, author={Li, Yanghao and Wang, Naiyan and Liu, Jiaying and Hou, Xiaodi}, booktitle={International Joint Conference on Artificial Intelligence}, year={2017} }
Results
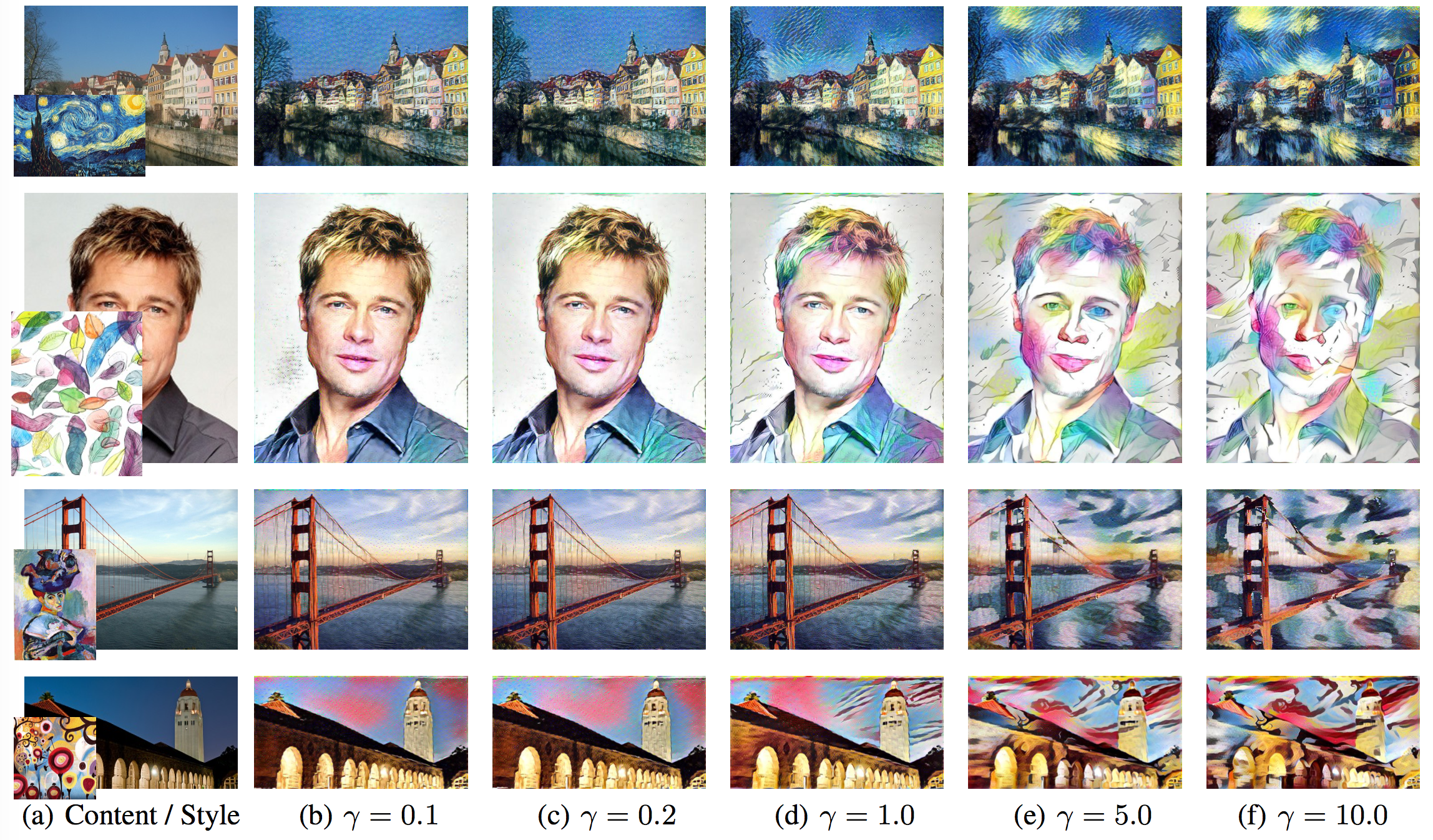
Fig. 2 Results of the four methods (linear, poly, Gaussian and BN) with different balance factor γ.
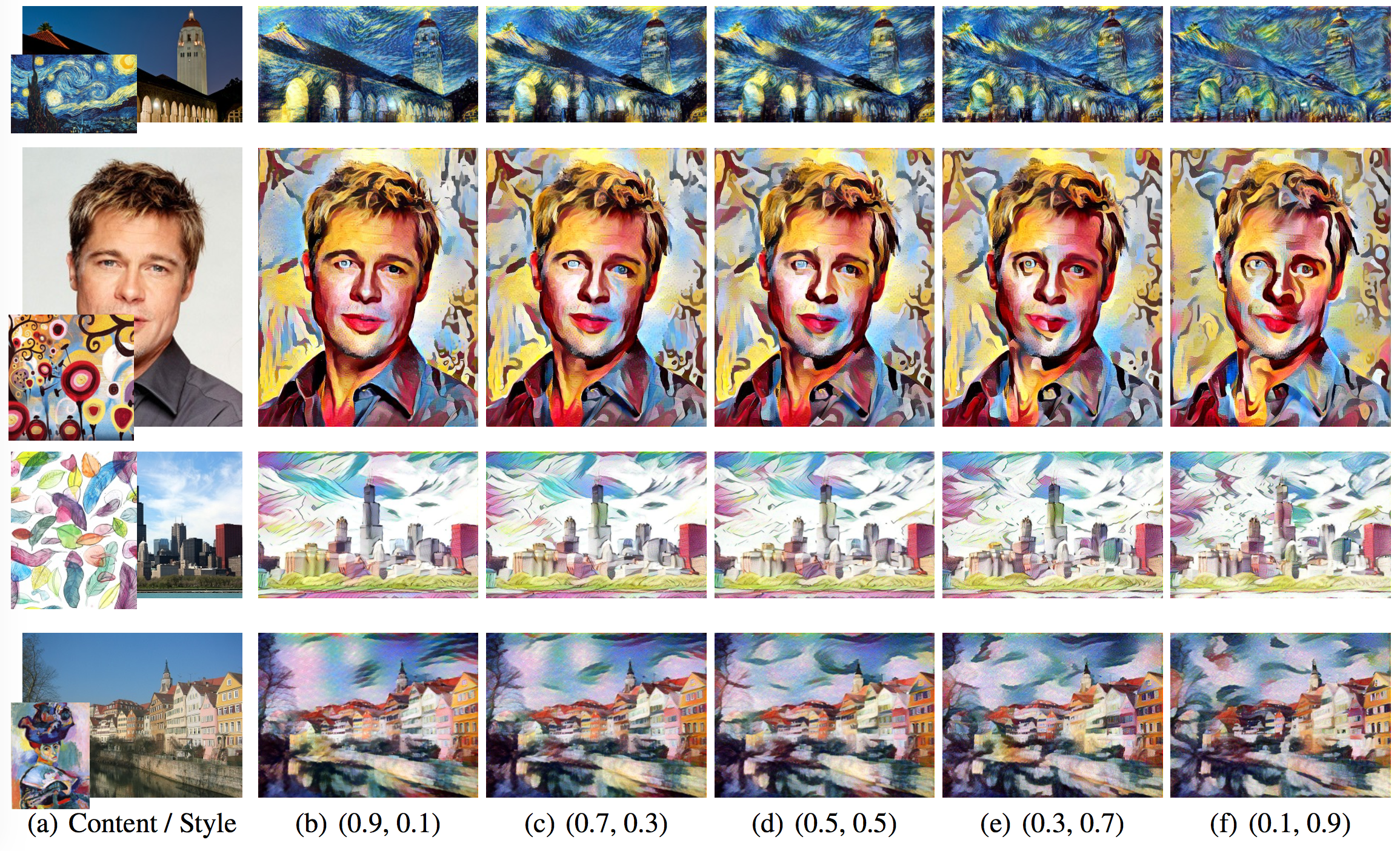
Fig. 3 Results of two fusion methods: BN + poly and linear + Gaussian.
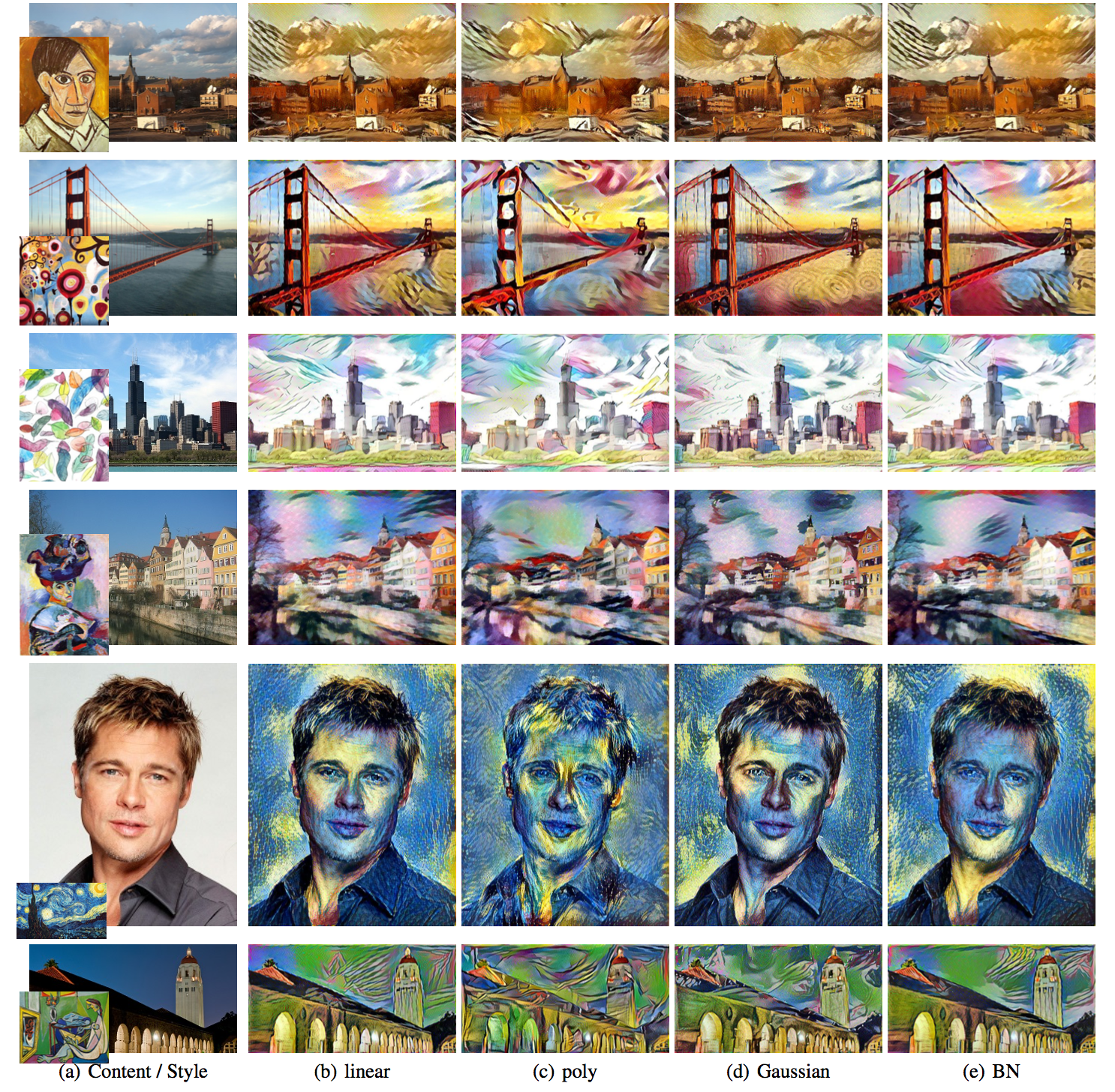
Fig. 4 Visual results of several style transfer methods, including linear, poly, Gaussian and BN.