Spatio-Temporal Attention-Based LSTM Networks for
3D Action Recognition and Detection
Abstract
Human action analytics has attracted a lot of attention for decades in computer vision. It is important to extract discriminative spatio-temporal features to model the spatial and temporal evolutions of different actions. In this work, we propose a spatial and temporal attention model to explore the spatial and temporal discriminative features for human action recognition and detection from skeleton data. We build our networks based on Recurrent Neural Networks (RNNs) with Long Short-Term Memory (LSTM) units. The learned model is capable of selectively focusing on discriminative joints of skeletons within each input frame and paying different levels of attention to the outputs of different frames. To ensure effective training of the network for action recognition, we propose a regularized cross-entropy loss to drive the learning process and develop a joint training strategy accordingly. \M{Moreover, based on temporal attention, we develop a method to generate action temporal proposals for action detection. We evaluate the proposed method on the SBU Kinect Interaction Dataset, the NTU RGB+D Dataset and the PKU-MMD Dataset, respectively. Experiment results demonstrate the effectiveness of our proposed model on both action recognition and action detection.
Action Recognition
Method & Results
For the detailed method and results, please refer to Spatio-Temporal Attention Model for Action Recognition (AAAI 2017)More Analysis
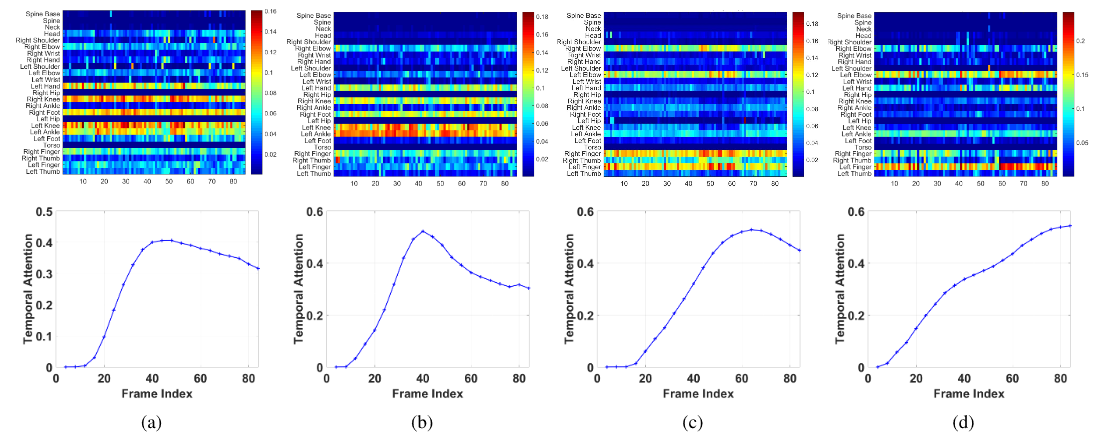
Fig.1 Visualization of spatial attention (top subfigures) and temporal attention (bottom subfigures) on actions of (a) sitting down, (b) kicking, (c) crossing hands, and (d) making a phone call (NTU-CS). Horizontal axis denotes the frame indexes (time).
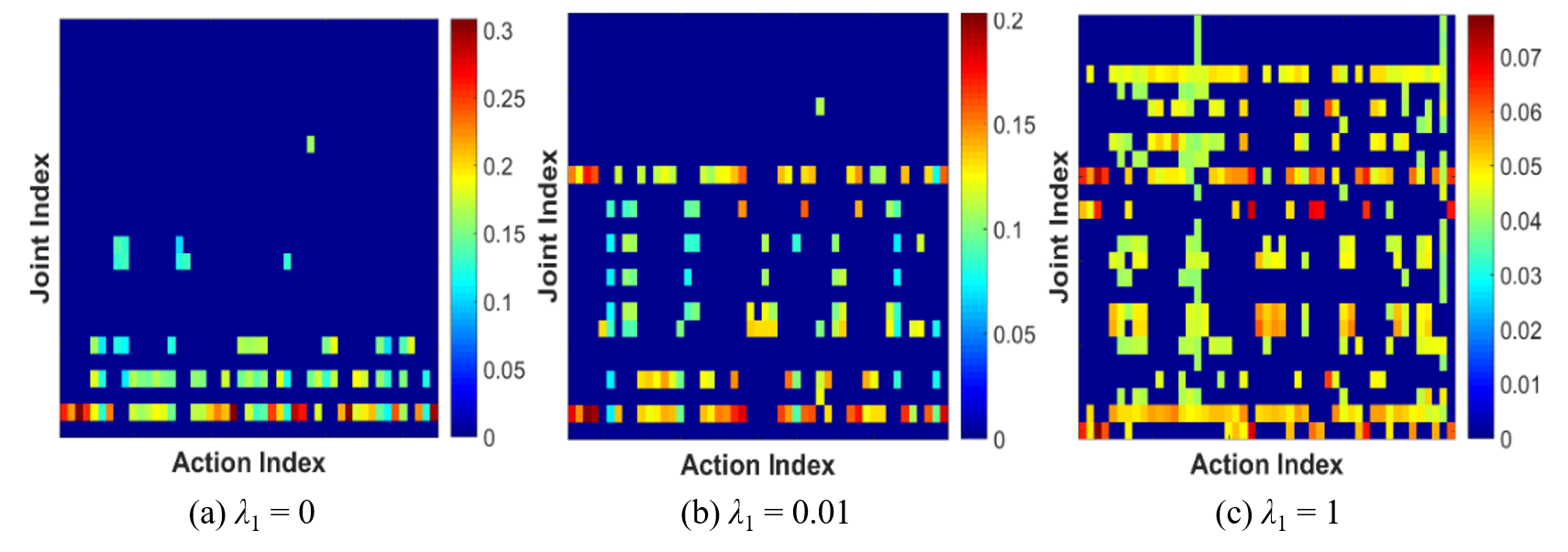
Fig.2 Distributionn of most engaged joints to different actions under various parameter settings (NTU-CS).
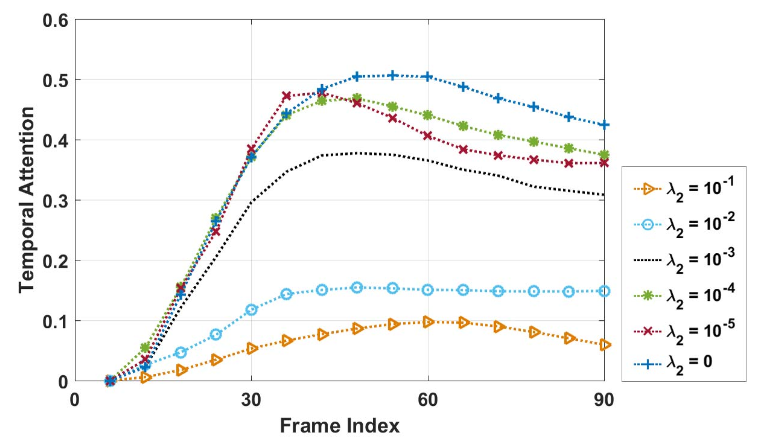
Fig.3 Temporal attention curves on action drinking water under different parameter settings.
Action Detection
Method
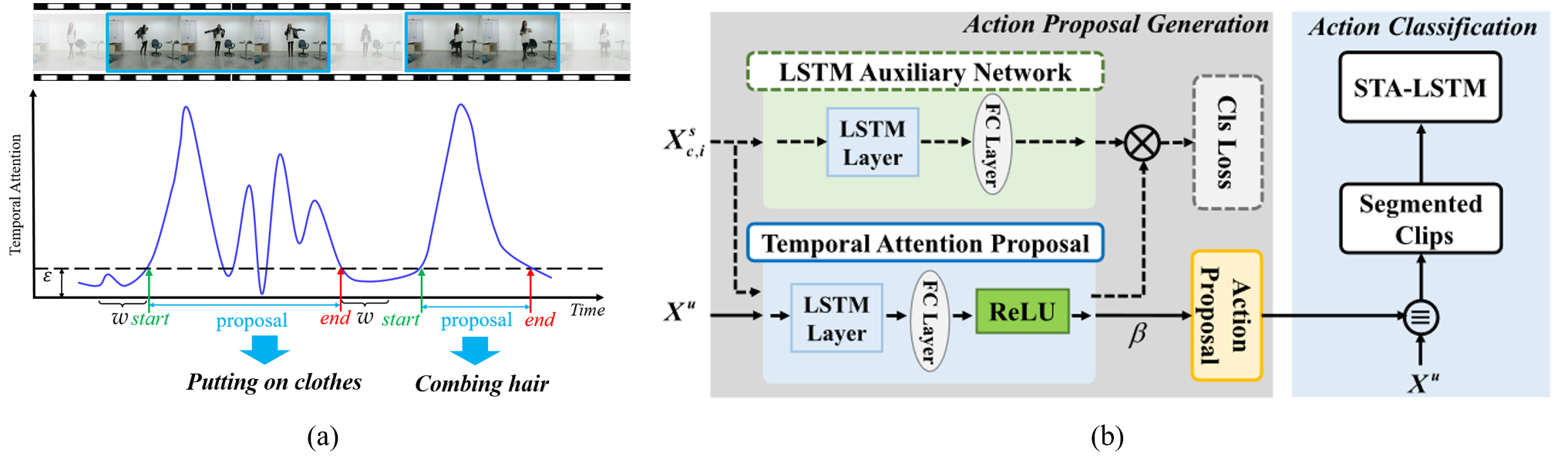
Fig.4 (a) Illustration for action detection (b) Architecture of the action detection framework.
Results
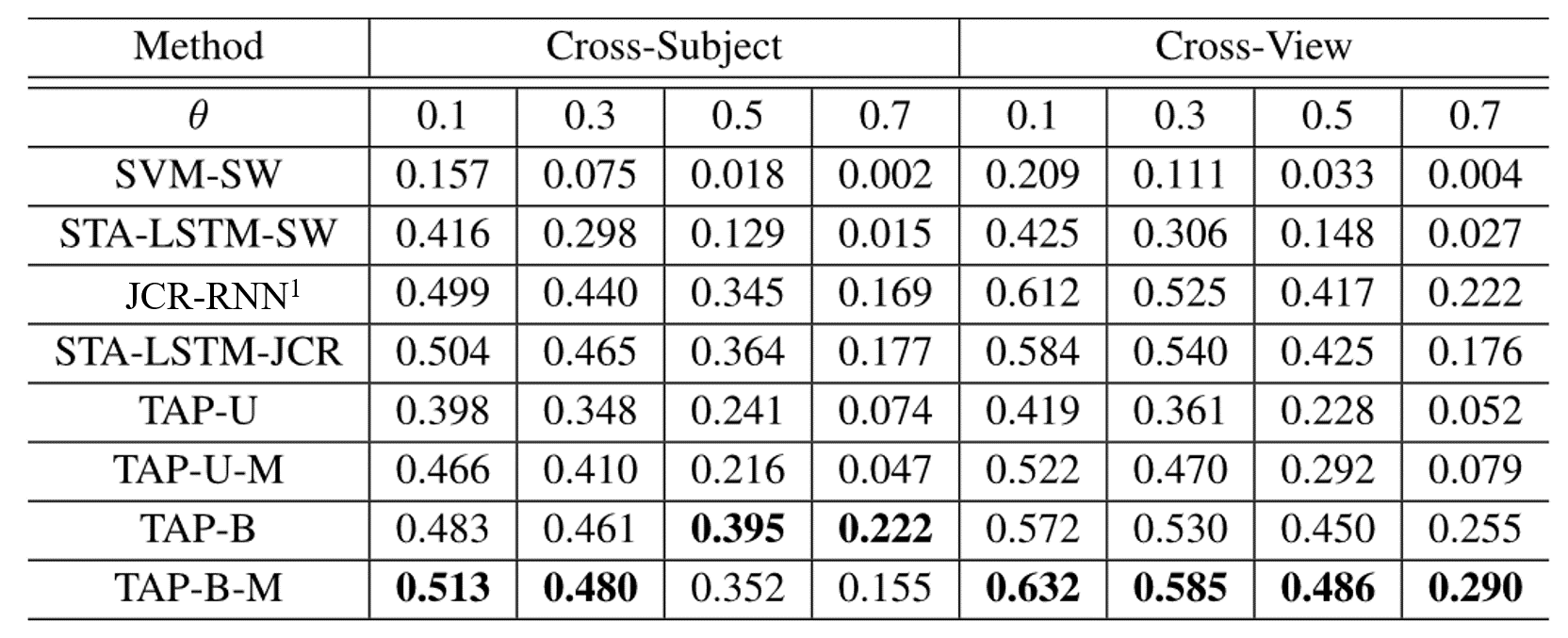
Table 1. Comparison of different methods in mAP on the PKUMMD.
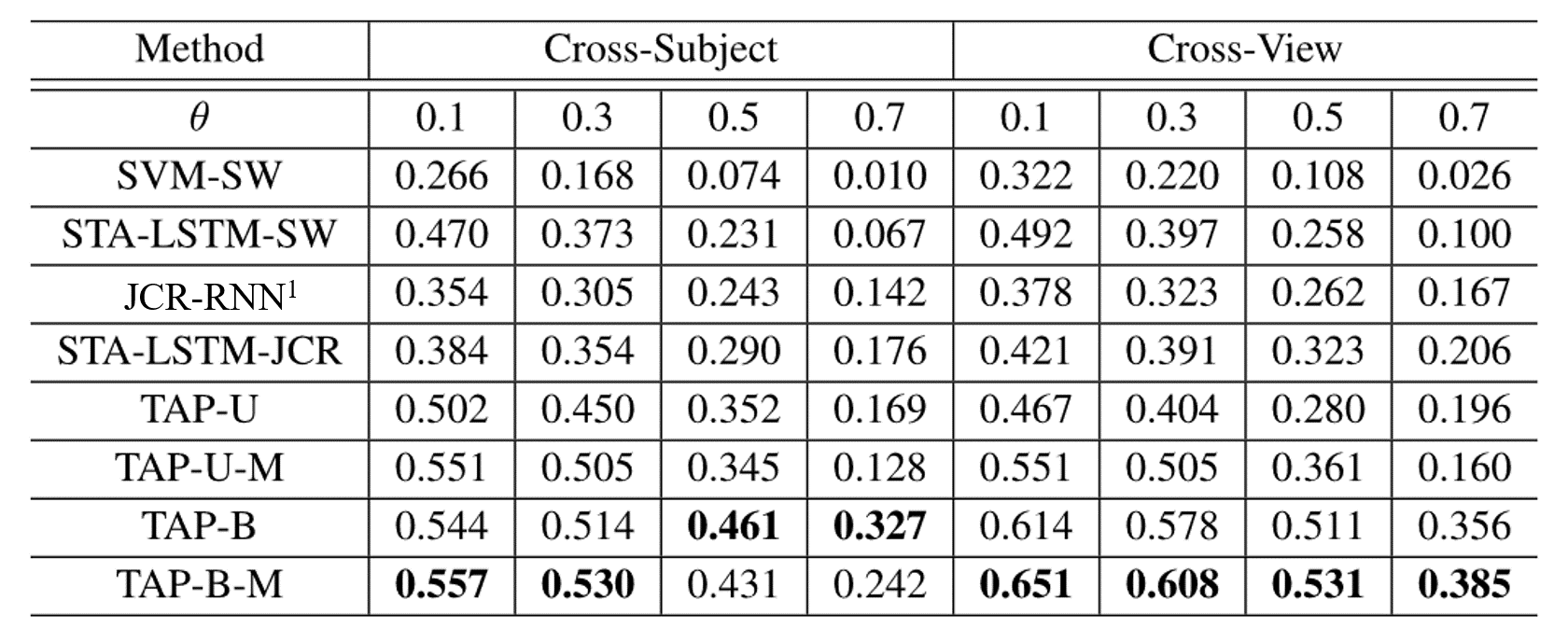
Table 2. Comparison of different methods in F1-Score on the PKUMMD.
Resources
Citation
@article{song2018spatio, title={Spatio-Temporal Attention-Based LSTM Networks for 3D Action Recognition and Detection}, author={Song, Sijie and Lan, Cuiling and Xing, Junliang and Zeng, Wenjun and Liu, Jiaying}, journal ={IEEE Transactions on Image Processing}, volume={27}, number={7}, pages={3459--3471}, year={2018} }
Reference
1. Y. Li, C. Lan, J. Xing, W. Zeng, C. Yuan, J. Liu. Online Human Action Detection using Joint Classification-Regression Recurrent Neural Networks. ECCV2016.