Video Super-Resolution Based on Spatial-Temporal Recurrent Neural Networks
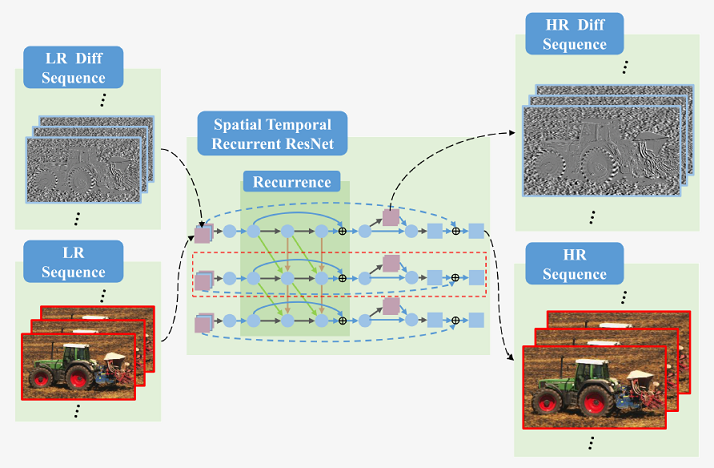
Fig. 1. The architecture of our proposed spatial-temporal recurrent residual network (STR-ResNet) for video SR. It takes not only the LR frames but also the differences of these adjacent LR frames as the input. Some reconstructed features are constrained to predict the differences of adjacent HR frames in the penultimate layer.
Abstract
In this paper, we propose a new video Super-Resolution (SR) method by jointly modeling intra-frame redundancy and inter-frame motion context in a unified deep network. Different from conventional methods, the proposed Spatial-Temporal Recurrent Residual Network (STR-ResNet) investigates both spatial and temporal residues, which are represented by the difference between a high resolution (HR) frame and its corresponding low resolution (LR) frame and the difference between adjacent HR frames, respectively. This spatial-temporal residual learning model is then utilized to connect the intra-frame and inter-frame redundancies within video sequences in a recurrent convolutional network and to predict HR temporal residues in the penultimate layer as guidance to benefit estimating the spatial residue for video SR. Extensive experiments have demonstrated that the proposed STR-ResNet is able to efficiently reconstruct videos with diversified contents and complex motions, which outperforms the existing video SR approaches and offers new state-of-the-art performances on benchmark datasets.
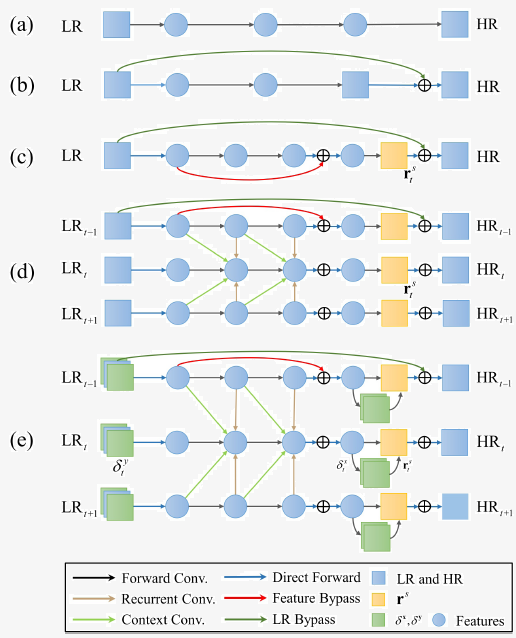
Fig. 2. Network architectures from vanilla SRCNN to our proposed spatialtemporal residual network. (a) SRCNN. (b) SRCNN with LR bypass connections. (c) SRes-CNN has both LR and feature bypass connections. (d) Multiple SRes-CNNs connected by context and recurrent convolutions to model inter-frame motion context. (e) In STR-ResNet, the differences of LR images are inputed into the network and parts of features in the penultimate layer aim to predict the differences of HR images, which further regularize and benefit the joint estimation of HR images.
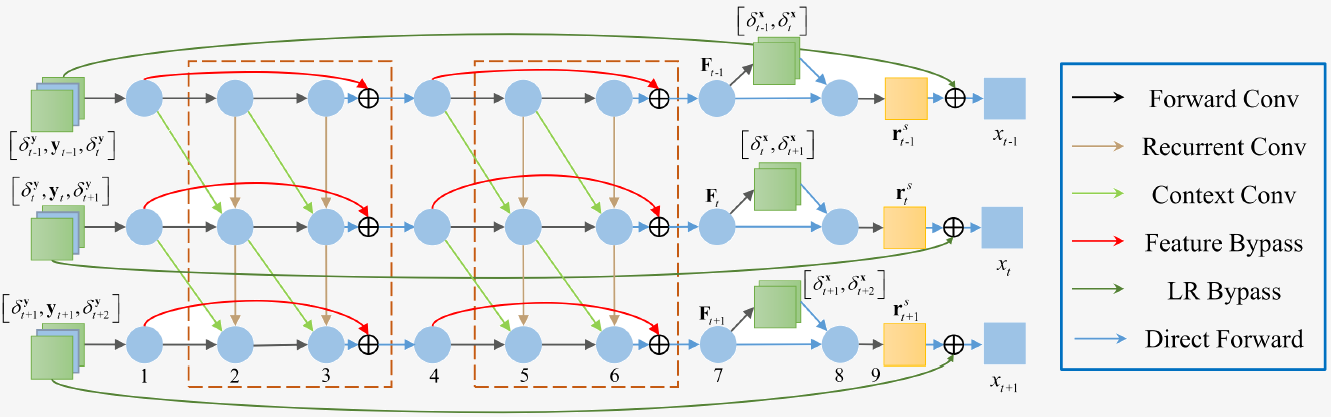
Fig. 3. The architecture of the STR-ResNet. It has a two-layer structure, which includes spatial and temporal residuals jointly in a unified deep framework. To model the inter-frame correlation, we construct a temporal residual RNN by piling up and connecting spatial residual CNNs. It takes not only the LR frames but also the differences of these adjacent LR frames as the input. Some reconstructed features are constrained to reconstruct the differences of adjacent HR frames in the penultimate layer.
Citation
@article{wenhan2017videoSR, title={Video Super-Resolution Based on Spatial-Temporal Recurrent Neural Networks}, author={Yang, Wenhan and Feng, Jiashi and Xie, Guosen and Liu, Jiaying Liu and Guo, Zongming and Yan, Shuicheng}, journal={Computer Vision and Image Understanding}, year={2018} }